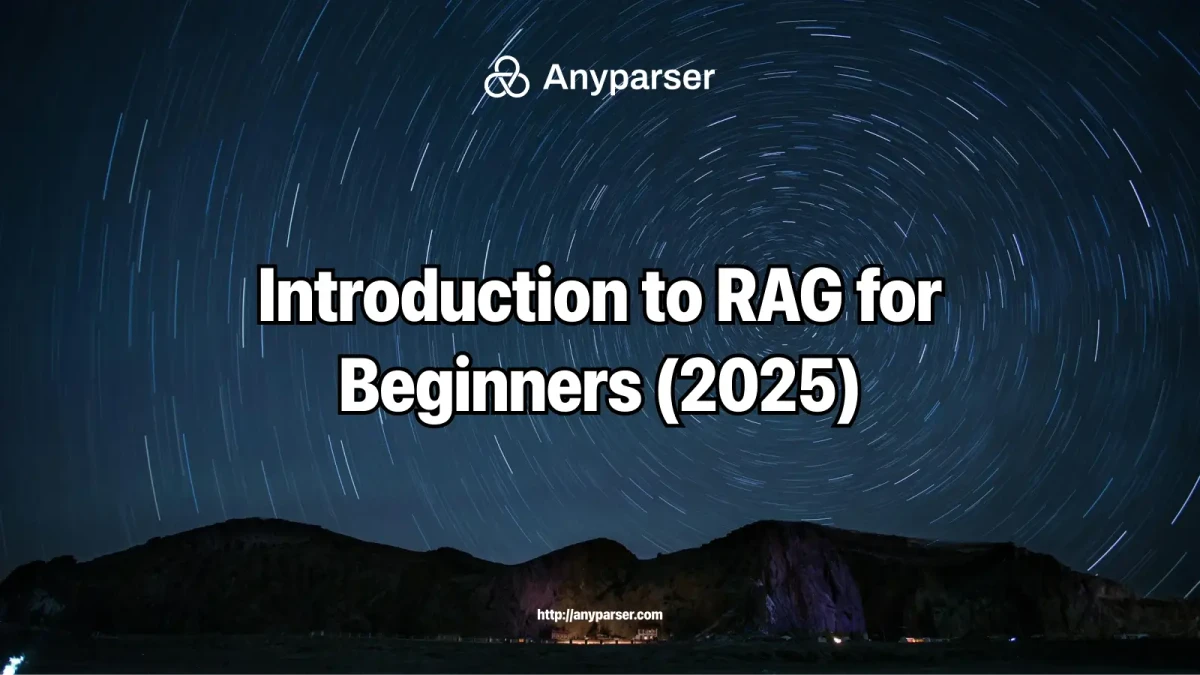
Understanding RAG: Introduction to RAG for Beginners (2025)
Explore Retrieval-Augmented Generation (RAG), its importance, and how it enhances AI accuracy. Learn about its applications and future.
Imagine if your favorite AI model could not only generate brilliant responses but also fetch the most up-to-date and relevant information in real-time. This is the magic of Retrieval-Augmented Generation (RAG)—a technique that’s transforming how AI interacts with data. If you’re familiar with AI, you know that large language models (LLMs) like GPT are incredibly smart. But what happens when these models encounter outdated or incomplete data? They’re limited by what they know, which, as we all know, isn’t always enough. That’s where RAG steps in.
In this blog post, we’ll explore how RAG works, why it’s a game-changer for AI, and how you can use it to stay ahead in an ever-changing world. Think of RAG as giving your AI a research assistant who never sleeps, always ensuring the information it provides is accurate, timely, and relevant.
What Is RAG? The Basics You Need to Know
To put it simply, Retrieval-Augmented Generation (RAG) is a hybrid approach that combines retrieval-based models (which fetch relevant data) with generative models (which generate human-like responses). It’s like asking your AI to not only come up with answers from its brain (its training data) but also to fetch the latest research, news, or documents from the web or a specific database.
Let’s break it down with a simple analogy. Imagine you’re writing a research paper, and you’re sitting in a library full of textbooks. You know a lot from what’s in the books, but there are also new journal articles and online resources that could add valuable insights. RAG is like hiring a research assistant who’s not only familiar with your textbooks but can also search the web, finding the latest publications and information to bring you the best answers possible.
Key Components of RAG:
- Retrieval-Based Models: These models search external databases for relevant data. Think of them as the “search engine” of your AI.
- Generative Models: Once the data is retrieved, these models process it and generate a cohesive, natural-sounding response.
So, rather than relying only on its built-in knowledge, RAG-equipped AI systems can fetch real-time information to improve their responses. This ability makes AI smarter and more accurate, especially in fast-moving industries where information changes constantly.
Why Does RAG Matter? The Benefits of Keeping AI Up-to-Date
1. Real-Time Access to Information
In a world where information is constantly evolving, relying on static data (data that doesn’t update after a model has been trained) simply doesn’t cut it anymore. With RAG, AI systems can access the most recent information available. Whether it’s news, research, or changes in regulations, RAG ensures your AI can offer insights based on the latest developments.
2. Increased Accuracy and Relevance
Traditional AI models, while impressive, can sometimes feel like they’re giving you textbook answers that might not be relevant anymore. With RAG, the AI is like a well-informed friend who not only knows the basics but also checks the current news to give you an up-to-the-minute response. This drastically improves the accuracy and relevance of the information it provides.
3. Improved User Experience
For users, this means better interactions. Imagine a healthcare chatbot that can pull up the latest research on a specific condition or a customer service bot that provides the most current product details. With RAG, users are more likely to receive quick, accurate, and helpful information.
How Does RAG Work? Behind the Scenes of AI’s New Power
Let’s take a closer look at how RAG blends the art of data retrieval with the craft of content generation.
-
Retrieving Data
First, the system uses a retrieval mechanism to pull in external information. This could be from a database, web search, or other online sources. It’s like going to the library and pulling up relevant articles or papers. -
Generating Content
Once the information is retrieved, the generative model takes over. It processes the data and integrates it with its existing knowledge to create a response. This is similar to how you might synthesize information from various sources and write a cohesive essay. -
Blending the Two
The magic of RAG happens when the retrieval and generation happen in tandem. It’s not just about finding data or generating text—it’s about combining the two to produce answers that are informed, accurate, and up-to-date.
Where Is RAG Used? Real-World Applications
RAG is already making waves in several industries, and its potential is only beginning to be realized. Here’s a glimpse of how RAG is transforming various sectors:
1. Healthcare: Saving Lives with Real-Time Research
Imagine you’re a doctor trying to make a quick diagnosis based on a patient’s symptoms. Using RAG, an AI can pull up the latest studies on similar conditions, offer potential diagnoses, and even suggest treatments—instantly. With the ability to access the latest medical research, RAG-equipped AI systems can help healthcare professionals make informed decisions quickly.
2. Customer Support: More Efficient, Smarter Chatbots
In customer service, RAG is enhancing chatbot capabilities. For example, when a customer asks about a product, the chatbot can immediately pull up the latest user manuals, product specs, or customer reviews from the company’s database, giving the customer the most relevant information possible. It’s like having a personal assistant who knows exactly where to look for answers.
3. Personalized Marketing: Tailoring Content to the Moment
Marketers can also use RAG to create highly personalized, contextually relevant campaigns. Imagine an AI generating a personalized email campaign that pulls in real-time data—like new product launches or current promotions—to make sure that the content is timely and directly related to a customer’s interests.
The Numbers Behind RAG: A Growing Market
The demand for smarter, more efficient AI is only growing. According to market research, the RAG market is expected to grow at an impressive rate of 44.7% annually from 2024 to 2030, reaching a value of over USD 1 billion by 2023. This rapid growth demonstrates just how pivotal RAG will be in the future of AI, with industries such as healthcare, e-commerce, and finance leading the charge in adoption.
Key Stats:
- 33.1% of global revenue in the RAG market came from document retrieval in 2023.
- Healthcare, finance, and retail are the leading sectors using RAG technologies.
- Augmented reality (AR) and virtual assistants are integrating with RAG for richer, more immersive user experiences.
Overcoming Challenges in RAG: The Roadblocks Ahead
As with any new technology, RAG is not without its challenges. While the benefits are clear, there are still hurdles to overcome:
1. Data Quality and Accuracy
One of the main concerns with RAG is the quality of the data being retrieved. If an AI pulls information from an unreliable or biased source, it could generate inaccurate or even harmful answers. Ensuring that the data is accurate, ethical, and free of bias is essential.
2. Integration Complexities
Integrating RAG into existing systems can be a challenge. It’s not just about plugging in a new model—it requires adapting current workflows, databases, and sometimes even retraining models. This can be time-consuming and expensive.
3. Data Privacy and Security
Given that RAG often involves accessing external data, ensuring that privacy and security standards are maintained is a top priority. Data breaches or mishandling of sensitive information could lead to significant issues, so robust security measures must be in place.
The Future of RAG: What’s Next?
Looking ahead, we can expect RAG to evolve into even more sophisticated systems. Experts predict multimodal RAG—AI systems that can not only work with text but also with images, video, and audio. This would mean AI could pull relevant information not just from documents but from multimedia sources as well.
Here are a few exciting possibilities:
- Personalized AI tutors that can access the latest educational materials and adjust their teaching methods based on the student’s progress.
- AI research assistants that can synthesize information from videos, podcasts, articles, and more, streamlining the research process across industries.
RAG will continue to push boundaries, making AI smarter, more adaptable, and even more integrated into our everyday lives.
The Role of Anyparser: Making RAG Work
Now, let’s talk about an exciting tool that’s essential for making RAG even more powerful—Anyparser.
Anyparser is a high-performance file conversion platform designed to simplify data extraction from a wide range of file types and URLs. It’s perfect for integrating data into RAG workflows. Imagine it as a tool that helps you extract useful, structured data from documents—whether it’s PDFs, Word files, or even media like videos and images.
Key Features of Anyparser:
- Wide Range of Supported Formats: PDFs, Word docs, Excel files, websites, audio, and more.
- Structured Data Output: Outputs in formats like Markdown and JSON, making it easy to integrate into your RAG systems.
- OCR Support: Extracts text from images and scanned documents, ensuring you don’t miss out on valuable data.
Why Anyparser is a Game-Changer:
Anyparser addresses critical challenges in the RAG ecosystem. Traditional document parsing tools are slow, expensive, and often inefficient. But Anyparser offers speed, accuracy, and **aff
ordability**—perfect for developers and enterprises looking to build sophisticated, data-rich AI systems.
Conclusion: The Future Is Now with RAG and Anyparser
Retrieval-Augmented Generation is more than just a buzzword—it’s reshaping how AI can provide real-time, relevant, and contextually accurate information. With the help of tools like Anyparser, integrating vast amounts of external data into your AI workflows has never been easier.
Are you ready to level up your AI game? Whether you’re in healthcare, finance, customer service, or marketing, RAG has the potential to supercharge your AI’s performance and relevance. And with Anyparser streamlining your data extraction, the future of AI is within your reach.
So, what are you waiting for? Dive into the world of Retrieval-Augmented Generation and see how it can transform your AI applications. The future of AI is here—and it’s smarter than ever.